In today’s dynamic e-commerce environment, personalization is no longer a luxury—it’s an expectation. Consumers are bombarded with choices, and what often makes a sale is not just what a brand offers, but how well it understands the customer. This is where AI-powered product recommendations step in.
From suggestions like “Frequently bought together” to “Recommended based on your past purchases,” AI-driven recommendation engines are reshaping the way online shoppers browse, engage, and buy. More than just a smart feature, AI recommendations are a key revenue driver, significantly increasing conversion rates, boosting average order values (AOV), and fostering long-term customer loyalty.
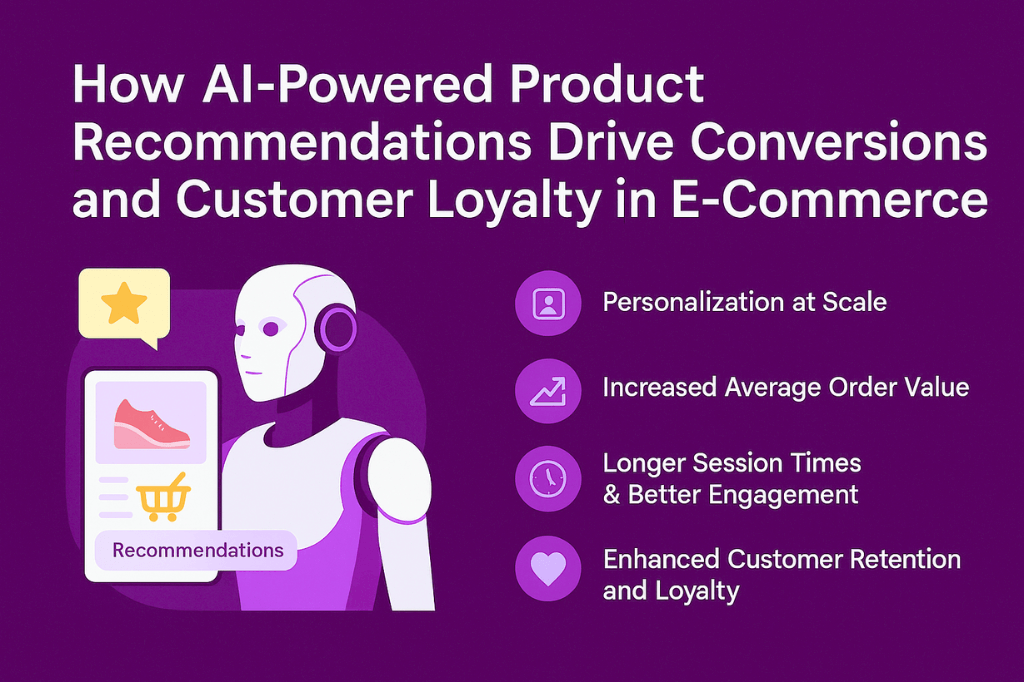
What Are AI-Powered Product Recommendations?
AI-powered product recommendations use machine learning algorithms, customer data, and real-time behavior analysis to suggest products that align with each shopper’s preferences and needs. These systems track how users interact with an online store—what they search for, click on, add to their cart, and buy—and use this data to offer contextual, relevant suggestions that feel uniquely tailored.
How Do AI Product Recommendations Work?
The magic of personalized recommendations lies in a multi-step process that turns customer data into high-impact suggestions:
1. Data Collection Across the Customer Journey
AI systems begin by collecting behavioral data across various touchpoints, such as:
- Search queries and site navigation
- Product page views and time spent
- Cart additions and wish lists
- Purchase history
- Device used (mobile, tablet, desktop)
This data paints a detailed picture of the shopper’s intent, preferences, and habits.
2. User Profiling and Pattern Recognition
Once enough data is gathered, AI builds a dynamic profile for each user. Using deep learning and pattern recognition, it can:
- Predict future behavior
- Identify product preferences
- Detect interests over time
These profiles are constantly updated to reflect the user’s evolving interests.
3. Real-Time Personalization with Machine Learning
Machine learning models then process all this input to generate real-time product recommendations. These models improve with use, learning from both individual and collective shopper behaviors to provide smarter, more accurate suggestions.
Read more about The Transformative Role of AI in E-commerce
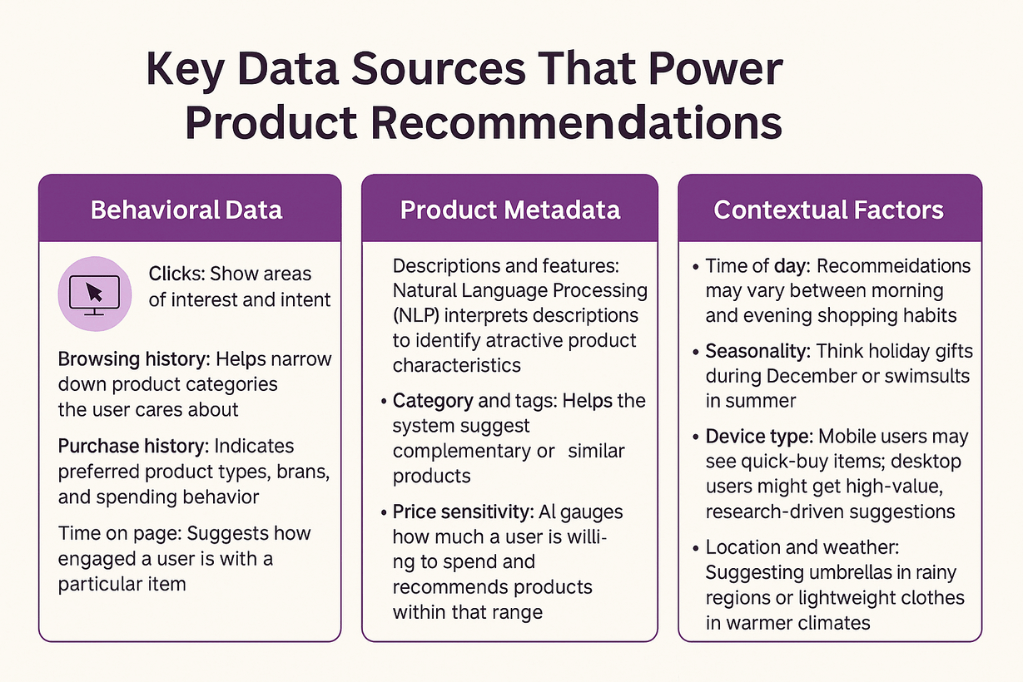
Key Data Sources That Power Product Recommendations
To ensure the recommendations are relevant and persuasive, AI analyzes several layers of data:
Behavioral Data
- Clicks: Show areas of interest and intent.
- Browsing history: Helps narrow down product categories the user cares about.
- Purchase history: Indicates preferred product types, brands, and spending behavior.
- Time on page: Suggests how engaged a user is with a particular item.
Product Metadata
- Descriptions and features: Natural Language Processing (NLP) interprets descriptions to identify attractive product characteristics.
- Category and tags: Helps the system suggest complementary or similar products.
- Price sensitivity: AI gauges how much a user is willing to spend and recommends products within that range.
- Stock availability: Ensures that recommendations reflect real-time inventory.
Contextual Factors
AI doesn’t operate in a vacuum. It also takes into account:
- Time of day: Recommendations may vary between morning and evening shopping habits.
- Seasonality: Think holiday gifts during December or swimsuits in summer.
- Device type: Mobile users may see quick-buy items; desktop users might get high-value, research-driven suggestions.
- Location and weather: Suggesting umbrellas in rainy regions or lightweight clothes in warmer climates can refine personalization further.
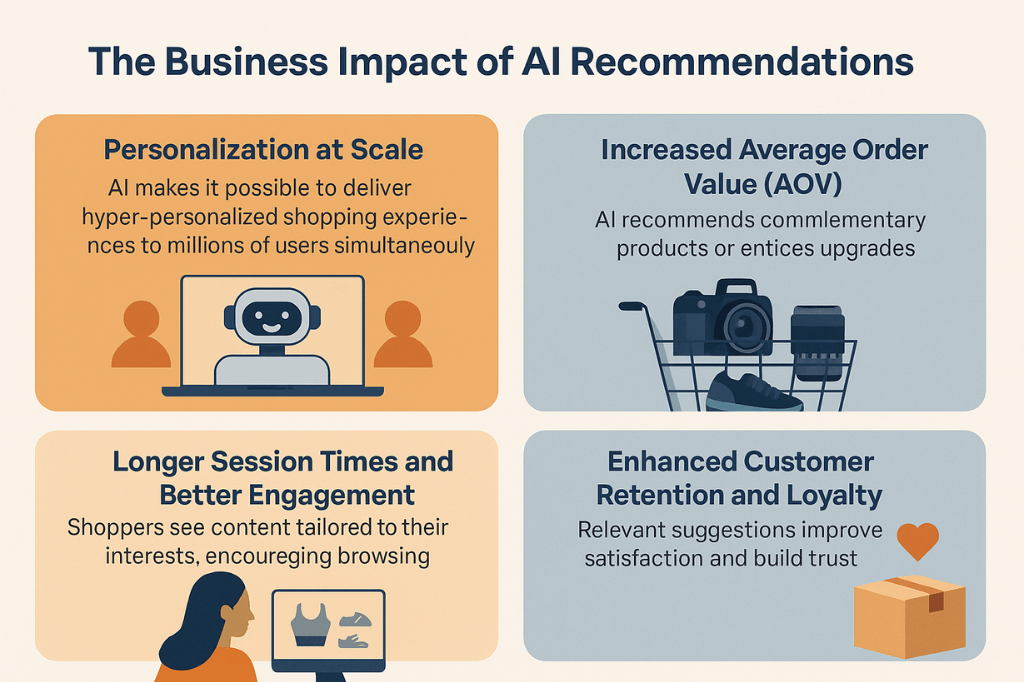
The Business Impact of AI Recommendations
1. Personalization at Scale
AI makes it possible to deliver hyper-personalized shopping experiences to millions of users simultaneously, which would be impossible through manual efforts. This level of personalization is proven to:
- Increase conversions
- Reduce bounce rates
- Encourage exploration and discovery
According to mckinsey, 76% of consumers get frustrated when digital experiences are not personalized. AI removes that friction by giving shoppers exactly what they want—even before they ask.
2. Increased Average Order Value (AOV)
By recommending complementary products or enticing upgrades, AI effectively implements cross-selling and upselling tactics. For example:
- A shopper viewing a camera might be shown lenses, tripods, and memory cards.
- Someone buying a shirt might get suggestions for matching pants and accessories.
This strategy increases the basket size without the customer feeling pressured—they perceive it as helpful guidance.
3. Longer Session Times and Better Engagement
When shoppers see content tailored to their interests, they naturally stay longer. They browse more, explore related products, and revisit items they didn’t notice before.
Let’s say someone is exploring a fitness apparel site. If they are shown gear that matches their preferred style or workout routine—such as yoga mats, resistance bands, or breathable tops—they’re more likely to interact, click through, and eventually buy.
4. Enhanced Customer Retention and Loyalty
AI doesn’t just convert—it helps keep customers coming back. Relevant suggestions improve satisfaction and build trust, reinforcing the feeling that your brand “gets” them.
Statistics show that 56% of shoppers are more likely to return to a site that recommends relevant products. The more relevant and consistent the experience, the higher the customer lifetime value (CLV).
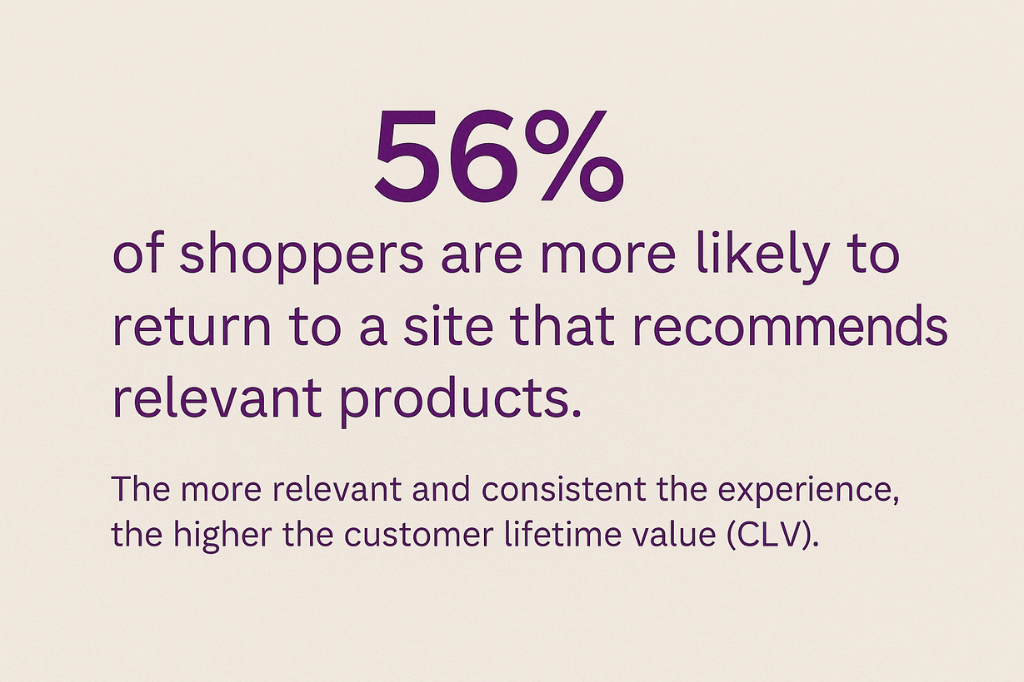
Real-Life Use Cases of AI-Powered Recommendations
Online Bookstores
AI analyzes users’ past reads and browsing behavior to recommend new titles. If a user loves fantasy novels, they might be shown books by related authors or trending titles in the same genre—effectively replicating a knowledgeable bookstore clerk.
Fashion Retailers
AI can curate full outfits based on what users view. A shopper exploring dresses might receive suggestions for shoes, bags, and jewelry that match the look—boosting both satisfaction and sales.
Subscription Services
Beauty boxes or food kits can be tailored based on a customer’s preferences and product reviews. AI helps determine which products should be included each month based on previous feedback and seasonal changes.
Continuous Learning and Optimization
AI recommendation engines are not static. They evolve with every click, scroll, and purchase. As users engage more, AI gets better at predicting their preferences. This continuous learning leads to:
- More accurate future suggestions
- Improved product curation
- Insights for merchandising and stock planning
Retailers can even discover unexpected purchasing trends and capitalize on them before competitors do.
Final Thoughts: Turn Browsers Into Buyers with AI
AI-powered product recommendations are not just a tech upgrade—they are a strategic growth tool. By creating relevant, engaging, and customized shopping experiences, they convert casual browsers into loyal buyers and maximize the value of every customer interaction.
Whether you’re running a startup store or managing a large e-commerce brand, AI personalization will give you a significant competitive edge in today’s crowded market.